
"Why PINN?"
Physics Informed Neural Networks:
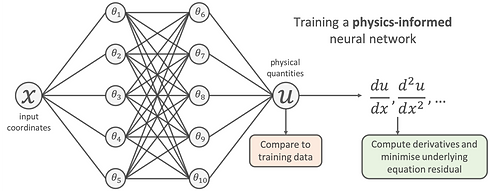
Physics-informed neural networks (PINNs) have gained popularity as a deep learning framework capable of blending observational data and partial differential equation (PDE) restrictions. This novel methodology has emerged as a multi-task learning framework in which an NN must fit observed data while reducing a PDE residual. Neural networks (NNs) that encode model equations, such as Partial Differential Equations (PDE), as a component of the neural network itself are called physics-informed neural networks (PINNs). In CDEI, PINN can be used in various cases as shown below.
Cloud Formation and Evolution
PINNs can simulate the formation and evolution of clouds by integrating known physical principles governing the behavior of water vapor condensation, cloud droplet formation, and cloud growth. It can be done by factoring in variables such as temperature, humidity, and atmospheric dynamics.
Radiative Transfer
Clouds have a significant impact on the Earth’s radiative balance by reflecting sunlight into space and trapping outgoing longwave radiation. PINNS can help in modeling radiative transfer within clouds, accounting for their varying optical properties, thickness, and altitude, contributing to a more accurate representation of their impact on energy balance.
Parameterization in Climate Models
PINNS offers a potential avenue to improve parametrization by capturing more detailed and physics-based representation of cloud behavior, reducing uncertainties in climate projections.
Cloud Microphysics
Understand the microphysical processes with clouds such as interactions between cloud particles, condensation, evaporation, and precipitation. PINNs will aid in modelling these processes by capturing complex relationships among various cloud constituents and environmental factors.
Cloud-Climate Feedback Loops
PINNS can assist in understanding and quantifying cloud-climate feedbacks by simulating how changes in cloud cover, type, or altitude affect atmospheric circulation, temperature, and precipitation patterns, thereby influencing the broader climate system.
Climate Model Validation
PINNS can be employed to validate existing climate models by comparing models outputs against observed cloud properties, helping identify areas where current models might be inaccurate or inadequate in representing cloud dynamics.
Predicting Extreme Weather Events
PINNs can aid in predicting the behavior and intensity of these events by simulating cloud-related processes and their interactions with larger atmospheric patterns.